Checking for non-preferred file/folder path names (may take a long time depending on the number of files/folders) ...
This resource contains some files/folders that have non-preferred characters in their name. Show non-conforming files/folders.
This resource contains content types with files that need to be updated to match with metadata changes. Show content type files that need updating.
Authors: |
|
|
---|---|---|
Owners: |
|
This resource does not have an owner who is an active HydroShare user. Contact CUAHSI (help@cuahsi.org) for information on this resource. |
Type: | Resource | |
Storage: | The size of this resource is 1.5 KB | |
Created: | Feb 08, 2023 at 2:37 p.m. (UTC) | |
Last updated: | Feb 08, 2023 at 2:38 p.m. (UTC) | |
Citation: | See how to cite this resource |
Sharing Status: | Public |
---|---|
Views: | 1181 |
Downloads: | 213 |
+1 Votes: | Be the first one to this. |
Comments: | No comments (yet) |
Abstract
Physically-based models of groundwater flow are powerful tools for water resources assessment under varying hydrologic, climate and human development conditions. One of the most important topics of investigation is how these conditions will affect the discharge of groundwater to rivers and streams (i.e. baseflow). Groundwater flow models are based upon discretized solution of mass balance equations, and contain important hydrogeological parameters that vary in space and cannot be measured. Common practice is to use least squares regression to estimate parameters and to infer prediction and associated uncertainty. Nevertheless, the unavoidable uncertainty associated with physically-based groundwater models often results in both aleatoric and epistemic model calibration errors, thus violating a key assumption for regression-based parameter estimation and uncertainty quantification. We present a complementary data-driven modeling and uncertainty quantification (DDM-UQ) framework to improve predictive accuracy of physically-based groundwater models and to provide more robust prediction intervals. First, we develop data-driven models (DDMs) based on statistical learning techniques to correct the bias of the calibrated groundwater model. Second, we characterize the aleatoric component of groundwater model residual using both parametric and non-parametric distribution estimation methods. We test the complementary data-driven framework on a real-world case study of the Republican River Basin, where a regional groundwater flow model was developed to assess the impact of groundwater pumping for irrigation. Compared to using only the flow model, DDM-UQ provides more accurate monthly baseflow predictions. In addition, DDM-UQ yields prediction intervals with coverage probability consistent with validation data. The DDM-UQ framework is computationally efficient and is expected to be applicable to many geoscience models for which model structural error is not negligible. (C) 2015 Elsevier Ltd. All rights reserved.
Subject Keywords
Coverage
Spatial
Content
Additional Metadata
Name | Value |
---|---|
DOI | 10.1016/j.cageo.2015.05.016 |
Depth | |
Scale | >100 000 km² |
Layers | 1 |
Purpose | Scientific investigation (not related to applied problem) |
GroMoPo_ID | 276 |
IsVerified | True |
Model Code | MODFLOW;Data Driven Algorithm |
Model Link | https://doi.org/10.1016/j.cageo.2015.05.016 |
Model Time | 1918-2000 |
Model Year | 2015 |
Model Authors | Xu, TF; Valocchi, AJ |
Model Country | United States |
Data Available | Report/paper only |
Developer Email | txu3@illinois.edu |
Dominant Geology | Unsure |
Developer Country | USA |
Publication Title | Data-driven methods to improve baseflow prediction of a regional groundwater model |
Original Developer | No |
Additional Information | A comparison of a MODFLOW model and a Data-driven approach for uncertainty analysis of groundwater flow modeling. |
Integration or Coupling | |
Evaluation or Calibration | Baseflow |
Geologic Data Availability | No |
How to Cite
This resource is shared under the Creative Commons Attribution CC BY.
http://creativecommons.org/licenses/by/4.0/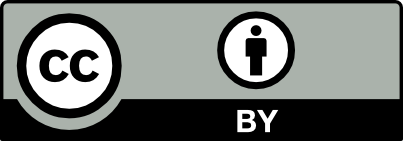
Comments
There are currently no comments
New Comment