Checking for non-preferred file/folder path names (may take a long time depending on the number of files/folders) ...
This resource contains some files/folders that have non-preferred characters in their name. Show non-conforming files/folders.
This resource contains content types with files that need to be updated to match with metadata changes. Show content type files that need updating.
Supporting data for publication (Prediction of flow duration curve for ungauged basins: Machine learning and deep learning approach)
Authors: |
|
|
---|---|---|
Owners: |
|
This resource does not have an owner who is an active HydroShare user. Contact CUAHSI (help@cuahsi.org) for information on this resource. |
Type: | Resource | |
Storage: | The size of this resource is 509.4 KB | |
Created: | Oct 24, 2024 at 2:10 a.m. | |
Last updated: | Oct 28, 2024 at 12:47 p.m. (Metadata update) | |
Published date: | Oct 28, 2024 at 12:47 p.m. | |
DOI: | 10.4211/hs.127d1b944fce46caaa89bac236627ed4 | |
Citation: | See how to cite this resource |
Sharing Status: | Published |
---|---|
Views: | 325 |
Downloads: | 6 |
+1 Votes: | Be the first one to this. |
Comments: | No comments (yet) |
Abstract
The flow duration curve characterizes streamflow variability, crucial for river management. Constructing FDCs is challenging in areas without gauging stations. This study explores machine learning and deep learning models, including random forest, deep neural network, support vector regression, and elastic net regression, to predict FDCs in ungauged basins. Using data from streamflow stations, we predict streamflow percentiles . The models utilize various combinations of accumulated precipitation and topographic features to improve prediction accuracy.
Subject Keywords
Content
How to Cite
This resource is shared under the Creative Commons Attribution CC BY.
http://creativecommons.org/licenses/by/4.0/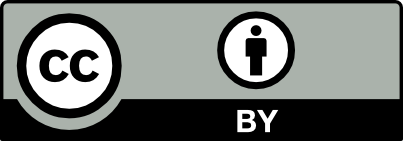
Comments
There are currently no comments
New Comment