Checking for non-preferred file/folder path names (may take a long time depending on the number of files/folders) ...
This resource contains some files/folders that have non-preferred characters in their name. Show non-conforming files/folders.
This resource contains content types with files that need to be updated to match with metadata changes. Show content type files that need updating.
Using Random Forest Classification to generate Better Wet Area Maps from High Resolution Digital Elevation Models: a Study in a Boreal Forest Landscape
Authors: |
|
|
---|---|---|
Owners: |
|
This resource does not have an owner who is an active HydroShare user. Contact CUAHSI (help@cuahsi.org) for information on this resource. |
Type: | Resource | |
Storage: | The size of this resource is 5.3 MB | |
Created: | Jun 04, 2018 at 8:30 a.m. (UTC) | |
Last updated: | Aug 15, 2018 at 1:09 p.m. (UTC) | |
Citation: | See how to cite this resource |
Sharing Status: | Public |
---|---|
Views: | 2655 |
Downloads: | 53 |
+1 Votes: | Be the first one to this. |
Comments: | 2 comments |
Abstract
Forested wetlands and wet soils near streams and lakes are often missing from current maps which makes wet areas difficult to manage. Wet soils also have lower bearing capacity making them more susceptible to soil disturbance from heavy forestry machines resulting in sediment and mercury transport to nearby surface waters. Topographical modelling of hydrological features such as topographical wetness index and depth to water has been suggested as a solution to this problem. These methods are easy to implement on large scales but are also static and do not take differences in spatial runoff patterns or soil textures into account. Therefore the aim of this study was to evaluate if machine learning can be used to create more accurate maps of wet soils. We used a machine learning approach where the National Forest Inventory of Sweden was used to train two random forest classifiers. The first model used a number of topographically derived variables along with soil types and runoff data while the second model only used topographically derived variables. The predicted maps were evaluated using Cohen’s kappa index of agreement, out of bag error and visual inspection of the Krycklan catchment. Both the predicted maps had a substantial agreement with the field plots and agreed well with the authors’ first-hand knowledge of the Krycklan catchment. Additionally there was sufficient information in the digital elevation model and including additional factors such as quaternary deposits and runoff only improved the overall accuracy by 1 % and increased kappa by 0.02.
Subject Keywords
Content
How to Cite
This resource is shared under the Creative Commons Attribution CC BY.
http://creativecommons.org/licenses/by/4.0/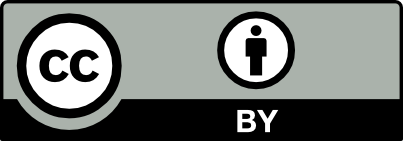
Comments
William Lidberg 7 years ago
The first collumn "ISWet" is the estimated wetness and the three last collumns "Taxar", "TraktNR", and "PalslagNR" is used to identify each plot.
ReplyWilliam Lidberg 7 years ago
The first collumn "ISWet" is the estimated wetness and the three last collumns "Taxar", "TraktNR", and "PalslagNR" is used to identify each plot.
ReplyNew Comment