Checking for non-preferred file/folder path names (may take a long time depending on the number of files/folders) ...
This resource contains some files/folders that have non-preferred characters in their name. Show non-conforming files/folders.
This resource contains content types with files that need to be updated to match with metadata changes. Show content type files that need updating.
Authors: |
|
|
---|---|---|
Owners: |
|
This resource does not have an owner who is an active HydroShare user. Contact CUAHSI (help@cuahsi.org) for information on this resource. |
Type: | Resource | |
Storage: | The size of this resource is 2.9 GB | |
Created: | Jun 17, 2024 at 5:11 p.m. | |
Last updated: | Aug 09, 2024 at 2:50 p.m. | |
DOI: | 10.4211/hs.4c8af0c89d2b4686808bcf9c6f7c0da3 | |
Citation: | See how to cite this resource |
Sharing Status: | Published |
---|---|
Views: | 205 |
Downloads: | 3 |
+1 Votes: | Be the first one to this. |
Comments: | No comments (yet) |
Abstract
The source is a repository of Spatially Explicit Estimate of Tile Drainage (SEETileDrain) products across the US Midwest in 2017 at a 30-m resolution. It includes the binary classification map (tile and non-tile), tile probability (how likely a grid cell is tile-drained). The Python scripts to generate the PAW layers and the R scripts (see also: https://github.com/LuwenWan/SEETileDrain_MidWest) to select variables, implement the random forest model and visualize the figures, are also available.
In this work, we developed a machine learning model using 31 satellite-derived and environmental variables and trained with 60,938 tile and non-tile ground truth points within the Google Earth Engine cloud computing platform. The results show that our model achieved good accuracy, with 96 % of the points correctly classified and an F1 score of 0.90. When the tile drainage areas are aggregated to the county scale, it agrees well (R-squared = 0.68) with the reported area from the 2017 Ag Census. The product, SEETileDrain (Spatially Explicit Estimate of Tile Drainage), is described in full detail in the manuscript and the supporting information of Wan et al. (2024). If needed, copies of the tile drainage product can be requested from the corresponding author at luven.wan@gmail.com.
Preferred citation:
L. Wan, A.D. Kendall, J. Rapp, D.W. Hyndman. 2024. Mapping agricultural tile drainage in the US Midwest using explainable random forest machine learning and satellite imagery, Science of the Total Environment. https://doi.org/10.1016/j.scitotenv.2024.175283
Subject Keywords
Coverage
Spatial
Temporal
Start Date: | |
---|---|
End Date: |
Content
Credits
Funding Agencies
This resource was created using funding from the following sources:
Agency Name | Award Title | Award Number |
---|---|---|
NASA | Linking Remote Sensing and Process-based Models to Better Understand the Influence of Land Use and Climate Changes on Great Lakes Coastal Wetlands | NNX11AC72G |
NASA | Integrating Systems Models and Remote Sensing to Explore Aquatic Ecosystem Vulnerability to Global Change in Lake Huron | 80NSSC21K1652 |
NOAA | Empowering Communities with Online Action Planning Tools: Tipping Points and Indicators for Improving Water Quality across the Great Lakes | NA12OAR4320071 |
NSF | Long-term Ecological Research Program at the Kellogg Biological Station and by Michigan State University AgBioResearch | DEB 2224712 |
CSC | Chinese Government Scholarship |
How to Cite
This resource is shared under the Creative Commons Attribution CC BY.
http://creativecommons.org/licenses/by/4.0/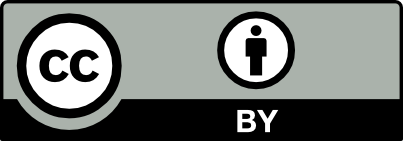
Comments
There are currently no comments
New Comment