Checking for non-preferred file/folder path names (may take a long time depending on the number of files/folders) ...
This resource contains some files/folders that have non-preferred characters in their name. Show non-conforming files/folders.
This resource contains content types with files that need to be updated to match with metadata changes. Show content type files that need updating.
Authors: |
|
|
---|---|---|
Owners: |
|
This resource does not have an owner who is an active HydroShare user. Contact CUAHSI (help@cuahsi.org) for information on this resource. |
Type: | Resource | |
Storage: | The size of this resource is 1.8 KB | |
Created: | Feb 08, 2023 at 2:45 p.m. | |
Last updated: | Feb 08, 2023 at 2:45 p.m. | |
Citation: | See how to cite this resource |
Sharing Status: | Public |
---|---|
Views: | 974 |
Downloads: | 216 |
+1 Votes: | Be the first one to this. |
Comments: | No comments (yet) |
Abstract
Bayesian inference using Markov Chain Monte Carlo (MCMC) provides an explicit framework for stochastic calibration of hydrogeologic models accounting for uncertainties; however, the MCMC sampling entails a large number of model calls, and could easily become computationally unwieldy if the high-fidelity hydrogeologic model simulation is time consuming. This study proposes a surrogate-based Bayesian framework to address this notorious issue, and illustrates the methodology by inverse modeling a regional MODFLOW model. The high-fidelity groundwater model is approximated by a fast statistical model using Bagging Multivariate Adaptive Regression Spline (BMARS) algorithm, and hence the MCMC sampling can be efficiently performed. In this study, the MODFLOW model is developed to simulate the groundwater flow in an arid region of Oman consisting of mountain-coast aquifers, and used to run representative simulations to generate training dataset for BMARS model construction. A BMARS-based Sobol' method is also employed to efficiently calculate input parameter sensitivities, which are used to evaluate and rank their importance for the groundwater flow model system. According to sensitivity analysis, insensitive parameters are screened out of Bayesian inversion of the MODFLOW model, further saving computing efforts. The posterior probability distribution of input parameters is efficiently inferred from the prescribed prior distribution using observed head data, demonstrating that the presented BMARS-based Bayesian framework is an efficient tool to reduce parameter uncertainties of a groundwater system. (C) 2018 Elsevier B.V. All rights reserved.
Subject Keywords
Coverage
Spatial
Content
Additional Metadata
Name | Value |
---|---|
DOI | 10.1016/j.jhydrol.2017.12.071 |
Depth | |
Scale | 1 001 - 10 000 km² |
Layers | 6 |
Purpose | Groundwater resources;Scientific investigation (not related to applied problem) |
GroMoPo_ID | 281 |
IsVerified | True |
Model Code | MODFLOW;Bayesian Multivariate Adaptive Regression Spline |
Model Link | https://doi.org/10.1016/j.jhydrol.2017.12.071 |
Model Time | 1993-2013 |
Model Year | 2018 |
Model Authors | Chen, MJ; Izady, A; Abdalla, OA; Amerjeed, M |
Model Country | Oman |
Data Available | Report/paper only |
Developer Email | cmj1014@gmail.com |
Dominant Geology | Model focuses on multiple geologic materials |
Developer Country | Oman |
Publication Title | A surrogate-based sensitivity quantification and Bayesian inversion of a regional groundwater flow model |
Original Developer | No |
Additional Information | Creating a Bayesian surrogate groundwater flow model and comparing it to a MODFLOW model of the wadi Al Fara in Oman. |
Integration or Coupling | None of the above |
Evaluation or Calibration | Dynamic water levels |
Geologic Data Availability | No |
How to Cite
This resource is shared under the Creative Commons Attribution CC BY.
http://creativecommons.org/licenses/by/4.0/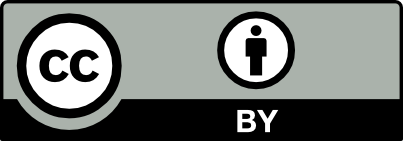
Comments
There are currently no comments
New Comment