Checking for non-preferred file/folder path names (may take a long time depending on the number of files/folders) ...
This resource contains some files/folders that have non-preferred characters in their name. Show non-conforming files/folders.
This resource contains content types with files that need to be updated to match with metadata changes. Show content type files that need updating.
Pretrained models + simulations for our HESSD submission "Towards learning universal, regional, and local hydrological behaviors via machine learning applied to large-sample datasets"
Authors: |
|
|
---|---|---|
Owners: |
|
This resource does not have an owner who is an active HydroShare user. Contact CUAHSI (help@cuahsi.org) for information on this resource. |
Type: | Resource | |
Storage: | The size of this resource is 895.7 MB | |
Created: | Jul 18, 2019 at 12:56 p.m. | |
Last updated: | Nov 12, 2021 at 4:48 p.m. (Metadata update) | |
Published date: | Dec 17, 2019 at 8:30 a.m. | |
DOI: | 10.4211/hs.83ea5312635e44dc824eeb99eda12f06 | |
Citation: | See how to cite this resource |
Sharing Status: | Published |
---|---|
Views: | 5216 |
Downloads: | 14266 |
+1 Votes: | 2 others +1 this |
Comments: | No comments (yet) |
Abstract
Contains all models trained for our publication "Towards learning universal, regional, and local hydrological behaviors via machine learning applied to large-sample datasets", as well as the evaluated model simulations. The set contains 48 runs in total, stemming from 3 different models (trained with 8 repetitions) and two different loss functions.
Subject Keywords
Content
README.md
Pretrained models and model simulations
Contains all models trained for our publication "Towards learning universal, regional, and local hydrological behaviors via machine learning applied to large-sample datasets", as well as the evaluated model simulations. The set contains 48 runs in total, stemming from 3 different models (trained with 8 repetitions) and two different loss functions.
About
The models are part of our manuscript "Towards learning universal, regional, and local hydrological behaviors via machine learning applied to large-sample datasets" https://arxiv.org/abs/1907.08456 that is accepted for publication in HESS.
Code
The code for the paper can be found here https://github.com/kratzert/ealstm_regional_modeling
Contact
Frederik Kratzer: kratzert@ml.jku.at
Related Resources
This resource is referenced by | Kratzert, F., Klotz, D., Shalev, G., Klambauer, G., Hochreiter S., and Nearing, G.: Towards learning universal, regional, and local hydrological behaviors via machine learning applied to large-sample datasets, Hydrol. Earth Syst. Sci., 2019. |
How to Cite
This resource is shared under the Creative Commons Attribution CC BY.
http://creativecommons.org/licenses/by/4.0/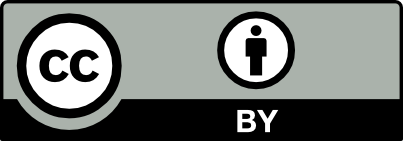
Comments
There are currently no comments
New Comment