Checking for non-preferred file/folder path names (may take a long time depending on the number of files/folders) ...
This resource contains some files/folders that have non-preferred characters in their name. Show non-conforming files/folders.
This resource contains content types with files that need to be updated to match with metadata changes. Show content type files that need updating.
Authors: |
|
|
---|---|---|
Owners: |
|
This resource does not have an owner who is an active HydroShare user. Contact CUAHSI (help@cuahsi.org) for information on this resource. |
Type: | Resource | |
Storage: | The size of this resource is 85.0 KB | |
Created: | Mar 26, 2024 at 7:45 p.m. | |
Last updated: | May 28, 2024 at 11:20 p.m. | |
Citation: | See how to cite this resource |
Sharing Status: | Public |
---|---|
Views: | 542 |
Downloads: | 36 |
+1 Votes: | 1 other +1 this |
Comments: | 1 comment |
Abstract
The increasing prevalence of nuisance benthic algal blooms in freshwater systems has led to water quality monitoring programs based on the presence and abundance of algae. Large blooms of the nuisance filamentous algae, Cladophora glomerata, have become common in the waters of the Upper Clark Fork River in western Montana. To aid in the understanding of algal growth dynamics, UAV-based hyperspectral images were gathered at three field sites along the length of the river throughout the growing season of 2021. Select regions within images covering the spectral range of 400-850 nm were labeled based on a combination of professional judgment and spectral profiles and used to train a random forest classifier to identify benthic algal growth across several classes, including benthic growth dominated by Cladophora (Clado), benthic growth dominated by growth forms other than Cladophora (non-Clado), and areas below a visually detectable threshold of benthic growth (bare substrate). After classification, images were stitched together to produce spatial distribution maps of each river reach while also calculating the average percent cover for each reach, achieving an accuracy of approximately 99% relative to manually labeled images. Results of this analysis showed strong variability across each reach, both temporally (up to 40%) and spatially (up to 46%), indicating that UAV-based imaging with high-spatial resolution could augment and therefore improve traditional measurement techniques that are spatially limited, such as spot sampling.
Subject Keywords
Coverage
Spatial
Temporal
Start Date: | |
---|---|
End Date: |
Content
How to Cite
This resource is shared under the Creative Commons Attribution-NoCommercial-ShareAlike CC BY-NC-SA.
http://creativecommons.org/licenses/by-nc-sa/4.0/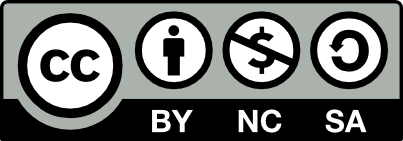
Comments
Riley Logan 10 months, 4 weeks ago
Please contact the authors for the hyperspectral images used to train the random forest classifier (they are too large to upload to HydroShare). Thanks for reading our work!
ReplyNew Comment