Checking for non-preferred file/folder path names (may take a long time depending on the number of files/folders) ...
This resource contains some files/folders that have non-preferred characters in their name. Show non-conforming files/folders.
This resource contains content types with files that need to be updated to match with metadata changes. Show content type files that need updating.
Authors: |
|
|
---|---|---|
Owners: |
|
This resource does not have an owner who is an active HydroShare user. Contact CUAHSI (help@cuahsi.org) for information on this resource. |
Type: | Resource | |
Storage: | The size of this resource is 174.8 MB | |
Created: | Apr 20, 2024 at 12:48 a.m. | |
Last updated: | Apr 24, 2024 at 6:13 a.m. | |
Citation: | See how to cite this resource | |
Content types: | Geographic Feature Content Geographic Raster Content |
Sharing Status: | Public |
---|---|
Views: | 742 |
Downloads: | 83 |
+1 Votes: | Be the first one to this. |
Comments: | No comments (yet) |
Abstract
This study investigates streamflow dynamics across contrasting climatic regions, focusing on arid Arizona, near-average California, and wet Michigan. The analysis integrates streamflow data from both USGS observational records and National Water Model forecasts for select stations within these regions. By examining these datasets, the study aims to provide insights into the hydrological behavior of diverse environments, elucidating the impacts of varying climatic conditions on streamflow patterns. Understanding these dynamics is essential for informed water resource management strategies tailored to the specific needs and vulnerabilities of each region.
Subject Keywords
Coverage
Spatial
Temporal
Start Date: | |
---|---|
End Date: |
Content
Data Services
Additional Metadata
Name | Value |
---|---|
Gage Type | River Gage |
Spatial data | GCS_WGS_1984 Projection |
NWM Forecasts | Time series data |
Analysis Periods | start=2021-04-21T00:00:00; end=2023-04-21T00:00:00 |
USGS Observation Streamflow | Time series data |
Related Resources
This resource is described by | Han, H., Morrison, R. R., (2022). Improved runoff forecasting performance through error predictions using a deep-learning approach. Journal of Hydrology (Elsevier) https://doi.org/10.1016/j.jhydrol.2022.127653 |
Credits
Funding Agencies
This resource was created using funding from the following sources:
Agency Name | Award Title | Award Number |
---|---|---|
Cooperative Institute for Research to Operations in Hydrology (CIROH) | Collaborative Research: Advancing Data Scienceand Analytics for Flood Predictions | None |
Contributors
People or Organizations that contributed technically, materially, financially, or provided general support for the creation of the resource's content but are not considered authors.
Name | Organization | Address | Phone | Author Identifiers |
---|---|---|---|---|
Daniel. P. Ames | Bigham Young University | Utah, US | (801) 422-3620 | ResearchGateID , GoogleScholarID |
How to Cite
This resource is shared under the Creative Commons Attribution CC BY.
http://creativecommons.org/licenses/by/4.0/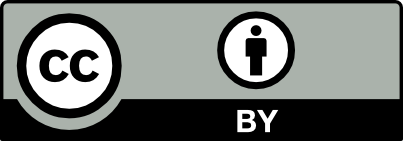
Comments
There are currently no comments
New Comment