Checking for non-preferred file/folder path names (may take a long time depending on the number of files/folders) ...
This resource contains some files/folders that have non-preferred characters in their name. Show non-conforming files/folders.
This resource contains content types with files that need to be updated to match with metadata changes. Show content type files that need updating.
Authors: |
|
|
---|---|---|
Owners: |
|
This resource does not have an owner who is an active HydroShare user. Contact CUAHSI (help@cuahsi.org) for information on this resource. |
Type: | Resource | |
Storage: | The size of this resource is 18.9 MB | |
Created: | Apr 24, 2024 at 10 p.m. | |
Last updated: | Apr 24, 2024 at 10:09 p.m. | |
Citation: | See how to cite this resource |
Sharing Status: | Public |
---|---|
Views: | 596 |
Downloads: | 4 |
+1 Votes: | Be the first one to this. |
Comments: | No comments (yet) |
Abstract
Snow distribution at wind-drift spatial scales ( 10 m) can be difficult to estimate due to modeling and observational constraints. Fortunately, the timing of snow disappearance is related to the distribution of snow water equivalent (SWE) throughout the spring snowmelt season. Here, we show that snow cover maps generated from PlanetScope’s constellation of Dove Satellites can resolve the 3 m date of snow disappearance across seven alpine domains in California and Colorado. Across a 5-year period (2019 – 2023), the average uncertainty in the date of snow disappearance, or the period of time between the last date of observed snow cover and first date of observed snow absence, was 3 days. Using a simple shortwave-based snowmelt model calibrated at nearby snow pillows, the PlanetScope date of snow disappearance could be used to reconstruct spring snow water equivalent (SWE). Relative to lidar SWE estimates, the SWE reconstruction had a spatial coefficient of correlation of 0.75, and SWE spatial variability that was biased by 9%, on average. SWE reconstruction biases were then improved to within 0.04 m, on average, by adjusting snowmelt rates using assumed distributions of SWE spatial heterogeneity and the evolution of fractional snow cover observed by PlanetScope. This study demonstrates the utility of fine-scale and high-frequency optical observations of snow cover, and the simple and annually repeatable connections between snow cover and spring snow water resources in regions with seasonal snowpack.
Subject Keywords
Coverage
Spatial
Temporal
Start Date: | |
---|---|
End Date: |
Content
README.txt
README file containing descriptions for data corresponding with Pflug et al. (2024) "Using commercial satellite imagery to reconstruct 3 m and daily spring snow water equivalent" Data last updated: J. Pflug (jpflug@umd.edu) - 24 April 2024 Data repository organization (capitalized entries = directories): - DAN: Dana Meadows, California (named after snow pillow ID, see publication Figure 1) - DPO: Devils Postpile, California - GIN: Gin Flat, California - STR: Ostrander Lake, California - 551: Joe Wright, Colorado - 737: Schofield Pass, Colorado - 869: Willow Creek Pass, Colorado - PILLOW (snow pillow snowmelt and shortwave radiation data for the simulation years) -(e.g., 2023) - MD.npy: Timestamp corresponding to the date of snowmelt onset used to calculate the relationship between cumulative radiation and cumulative snowmelt (in python numpy array-readable format: .npy) - meltSeason_SW.npy: daily-average shortwave radiation (W/m^2) from the snow pillow site, 364 days starting 1 October of the water year (e.g., 1 October 2022 for water-year 2023) - MF: - Entry 1: Melt Factor (MF, m^3/W, Equation 1 in the manuscript) - Entry 2: fitting parameter (C, m, Equation 1) - PS_PROCESSED (data processed from Raw PlanetScope imagery. Raw imagery not allowed to be shared, per data agreement) - snow_classes.tif: 4 unique snow classes classified based on equal-area regions with annual-average snow disappearance timing, ranging from early snow disappearance (0) to late snow persistence (3). Data is georeferenced in tiff format. - 3class_model.joblib: Random-forest model used to derive snow presence, snow absence, and image artifacts from the raw PlanetScope imagery - (e.g., 2023) - SCA_stacked.npy: 3 dimensional array (time,y,x) of binary snow cover (1) and absence (0) derived from PS imagery. y and x coordinates correspond with dimensions in the georeferenced snow class tiffs (snow_classes.tif) - SCA_WYdates.npy: array of dates, defined as the number of days since the start of the water year (1 October) corresponding to the time dimension of 'SCA_stacked.npy' - SWEMap_Method<#>_ .npy: SWE predictions made using the three modeling methods detailed by Pflug et al (2024). SWE predictions are on dates corresponding with lidar observations (in year month day format: yyyymmdd)
Credits
Funding Agencies
This resource was created using funding from the following sources:
Agency Name | Award Title | Award Number |
---|---|---|
NASA | A.44 Commercial Smallsat Data Scientific Analysis | 80NSSC24K0050 |
How to Cite
This resource is shared under the Creative Commons Attribution CC BY.
http://creativecommons.org/licenses/by/4.0/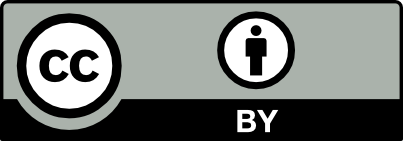
Comments
There are currently no comments
New Comment