Checking for non-preferred file/folder path names (may take a long time depending on the number of files/folders) ...
This resource contains some files/folders that have non-preferred characters in their name. Show non-conforming files/folders.
This resource contains content types with files that need to be updated to match with metadata changes. Show content type files that need updating.
Combining Climate Forcings and Initial Hydrological Conditions for Enhanced Subseasonal-to-Seasonal Flood Forecasting
Authors: |
|
|
---|---|---|
Owners: |
|
This resource does not have an owner who is an active HydroShare user. Contact CUAHSI (help@cuahsi.org) for information on this resource. |
Type: | Resource | |
Storage: | The size of this resource is 1.6 GB | |
Created: | Apr 12, 2024 at 2:26 a.m. | |
Last updated: | Jan 28, 2025 at 6:35 a.m. | |
Citation: | See how to cite this resource | |
Content types: | Multidimensional Content |
Sharing Status: | Public |
---|---|
Views: | 272 |
Downloads: | 0 |
+1 Votes: | Be the first one to this. |
Comments: | No comments (yet) |
Abstract
This study enhances the understanding of the predictability of subseasonal-to-seasonal (S2S) scale streamflow and flooding and their forecasting skills, while tackling long-time challenges introduced by low-skill climate forcings (SCFs). Utilizing six numerical weather prediction (NWP) models and a calibrated hydrological model, we assess the precipitation, streamflow, and flood predictability at 24 river stations in the rainfall-dominant Pearl River Basin, South China. Various model configurations are conducted to address uncertainties in initial hydrological conditions (IHCs) and SCFs, with the conventional ensemble streamflow prediction (ESP) serving as the benchmark. The findings are: (1) NWP driven hydrological forecasts demonstrate enhanced streamflow forecasting proficiency, achieving KGE >0.5 over 44 days, significantly outperforming its precipitation forecast skill with KGE >0.2 up to 10 days; (2) NWP-based deterministic streamflow predictions also outperform ESP, with KGE exceeding 0.6 for 20 days versus ESP's 5 days, and the Critical Success Index (CSI) for flood event detection over 0.3 for three weeks compared to only one week for ESP; (3) Bias correction of NWP precipitation further improves deterministic streamflow forecasts, with KGE > 0.6 for 30 days while having less effects on probilistic forecasting; (4) The performance enhancement from precipitation to streamflow forecast is mainly due to the persistent and slow response of streamflow to subsurface flow, while it is dominated by baseflow condition. Overall, IHCs initially dominate S2S forecasting, while SCF's impact accumulates, overtaking IHCs at a certain lead time, which indicates a future pathway to shift the S2S "predictability desert" narrative by enhancing both IHC and SCF.
Subject Keywords
Coverage
Spatial
Temporal
Start Date: | |
---|---|
End Date: |
Content
Data Services
How to Cite
This resource is shared under the Creative Commons Attribution CC BY.
http://creativecommons.org/licenses/by/4.0/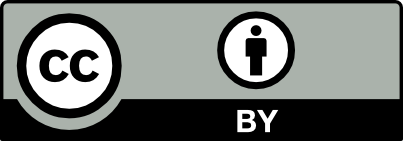
Comments
There are currently no comments
New Comment