Checking for non-preferred file/folder path names (may take a long time depending on the number of files/folders) ...
This resource contains some files/folders that have non-preferred characters in their name. Show non-conforming files/folders.
This resource contains content types with files that need to be updated to match with metadata changes. Show content type files that need updating.
Authors: |
|
|
---|---|---|
Owners: |
|
This resource does not have an owner who is an active HydroShare user. Contact CUAHSI (help@cuahsi.org) for information on this resource. |
Type: | Resource | |
Storage: | The size of this resource is 1.1 MB | |
Created: | Dec 11, 2017 at 5:55 a.m. (UTC) | |
Last updated: | Jan 24, 2018 at 6:12 p.m. (UTC) (Metadata update) | |
Published date: | Jan 24, 2018 at 6:12 p.m. (UTC) | |
DOI: | 10.4211/hs.a2661d94a39e449bad34663c32cf485c | |
Citation: | See how to cite this resource |
Sharing Status: | Published |
---|---|
Views: | 3541 |
Downloads: | 85 |
+1 Votes: | Be the first one to this. |
Comments: | No comments (yet) |
Abstract
The Arkavathy watershed in southern India has exhibited considerable changes in inflow to major reservoirs, but explanations for these changes have been hampered by limited hydrological records in the watershed. In order to understand long-term hydrological change in this heavily managed watershed, we developed a spatially distributed dataset of surface water by generating time series of water extent in nearly 1700 man-made lakes, or tanks, over a 40-year period. Using an automated classification approach with subpixel unmixing, we classified water extent in each of the tanks in 40 Landsat images from 1973 to 2010, including 16 end-of-monsoon-season (December or January) images which characterized tank status after the rainy season. An additional 8 images in 2013 and 2014 were classified to analyze dry-season behavior and for validation. The classification results compared well with a reference dataset of water extent of tanks (R-squared=0.95). We also evaluated hydrological change in the watershed in 42 clusters of tanks by modeling water extent in the cluster on hydrological covariates and time. Based on a water balance argument, we inferred that any statistically significant results for the coefficient on the time covariate were indicative of long-term hydrological change. The results of this remote sensing classification for each of the tanks in the Arkavathy watershed are contained in this resource. The covariates and results from the statistical model are also included.
Subject Keywords
Content
readme.txt
This dataset contains water extent (surface water area) of tanks in the Arkavathy watershed in Karnataka, India. The dataset was developed as part of a research project to understand hydrological change in the Arkavathy watershed from 1973 to 2010. Long-term trends in water extent in clusters of tanks are also included. The details of the classification algorithm can be found in Penny et al. (2018), along with accuracy assessment of the dataset. The classification was validated using a reference dataset, with coefficient of determination of 0.95 for all of the tanks. Tanks with larger water extent were generally predicted more accurately, while tanks with small water extent were not predicted as accurately. Errors were unbiased, so that aggregating multiple tanks tended to increase the accuracy of the aggregated area. TankWaterExtent.csv: For each tank in each scene, the tank water extent is given in square meters. Column headers indicate the Landsat ID, which can be matched with the ID in TankMeta.csv. It contains the Landsat mission and date of the image. Negative numbers in the area field indicate error codes. See below for a description of error codes and reason for omission. TankMeta.csv: Contains metadata for each of the tanks. TankID # ID number for the tank or reservoir. lat, lon # latitude and longitude, at the center of the tank name # reservoir name, if any. Tanks are left NA. ClusterID # ID number of the cluster into which each of the tanks was aggregated for analysis. Reservoirs are grouped into Cluster 0 (Byramangala was excluded due to the unreliability of classification due to algae blooms). LandsatMeta.csv: Contains metadata for each of the Landsat scenes. SceneID # Landsat scene ID, which matches with the column headers in TankWaterExtent.csv date # date of the image capture mission # Landsat mission sensor # Landsat sensor path, row # path and row of the image. MSS images use WRS-1, while all other images are referenced using WRS-2 data_source # most images were obtained from USGS, but some were obtained from NRSC cloud_free # images marked as “cloud free” contained no clouds, and the cloud detection algorithm was not run. ClusterCovariates.csv: Contains the covariates with which to model hydrological change for each cluster. ClusterID # ID number of the cluster into which each of the tanks was aggregated for analysis. date # Date of the Landsat image, in the end-of-monsoon period (December-January). WaterAreaFraction # Aggregate water area divided by the aggregate maximum water area for the cluster. Set to NA only if the entire cluster was outside of the Landsat scene boundary. MonsoonYear # The year of the monsoon season preceding the Landsat image. P_total # Total precipitation from September 1 to the date of the image. P_extreme # Average precipitation in large storms from September 1 to the date of the image. DSD # Dry-season days, or the number of days after December 01. Flag # 0 indicates no flag. 1 indicates that the some tanks in the cluster had errors (indicated by error codes in TankWaterExtent.csv), and the aggregate maximum area of these tanks was at least 30% of the aggregate maximum area of all tanks in the cluster. StatsModelResults.csv: Contains the results of the statistical model. Watershed # Name of the cluster watershed, including the Cluster ID. Area_km2 # Total area of the cluster watershed. Predictor # The category of covariate in the model. Coefficient # The coefficient designation for the covariate. Estimate # The estimated value of the coefficient (based on the centered data). Conf_Interval # 95% confidence interval around the estimate of the coefficient. Significant # Statistical significance of the trend. If "Y", the 95% confidence interval excluded 0. Max_extent_ha # Sum of the maximum water extent of each tank within the cluster. Trend_ha_per_10km2_decade # Long-term (residual) trend detected from the statistical analysis, in units of ha per 10 sq. km per decade. ClusterID # ID number of the cluster. ClusterWatersheds.zip: Compressed folder containing ClusterWatersheds.shp, a shapefile of cluster watersheds, including Cluster IDs and Subcatchment names. Penny, G., Srinivasan, V., Dronova, I., Lele, S., & Thompson, S. (2018). Spatial characterization of long-term hydrological change in the Arkavathy watershed adjacent to Bangalore, India. Hydrology and Earth System Sciences, 22, 595–610. http://doi.org/10.5194/hess-22-595-2018 Error codes (negative numbers in TankWaterExtent.csv): SLC off overlap, along with: -81 # Some water in tank -82 # Cloud/shadow overlap -91 # Dry tank -92 # Dry & cloud/shadow overlap MSS NA overlap, along with -75 # Complete overlap -85 # Some water in tank -86 # Cloud/shadow overlap -95 # Dry tank Cloud/shadow overlap only, along with -89 # Some water in tank -99 # Dry tank
How to Cite
This resource is shared under the Creative Commons Attribution CC BY.
http://creativecommons.org/licenses/by/4.0/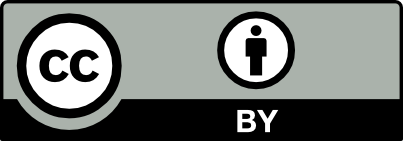
Comments
There are currently no comments
New Comment