Checking for non-preferred file/folder path names (may take a long time depending on the number of files/folders) ...
This resource contains some files/folders that have non-preferred characters in their name. Show non-conforming files/folders.
This resource contains content types with files that need to be updated to match with metadata changes. Show content type files that need updating.
Authors: |
|
|
---|---|---|
Owners: |
|
This resource does not have an owner who is an active HydroShare user. Contact CUAHSI (help@cuahsi.org) for information on this resource. |
Type: | Resource | |
Storage: | The size of this resource is 1.5 KB | |
Created: | Feb 08, 2023 at 4:09 p.m. | |
Last updated: | Feb 08, 2023 at 4:10 p.m. | |
Citation: | See how to cite this resource |
Sharing Status: | Public |
---|---|
Views: | 851 |
Downloads: | 213 |
+1 Votes: | Be the first one to this. |
Comments: | No comments (yet) |
Abstract
Pumping optimization of coastal aquifers involves complex numerical models. In problems with many decision variables, the computational burden for reaching the optimal solution can be excessive. Artificial Neural Networks (ANN) are flexible function approximators and have been used as surrogate models of complex numerical models in groundwater optimization. However, this approach is not practical in cases where the number of decision variables is large, because the required neural network structure can be very complex and difficult to train. The present study develops an optimization method based on modular neural networks, in which several small subnetwork modules, trained using a fast adaptive procedure, cooperate to solve a complex pumping optimization problem with many decision variables. The method utilizes the fact that salinity distribution in the aquifer, depends more on pumping from nearby wells rather than from distant ones. Each subnetwork predicts salinity in only one monitoring well, and is controlled by relatively few pumping wells falling within certain control distance from the monitoring well. While the initial control area is radial, its shape is aclaptively improved using a Hermite interpolation procedure. The modular neural subnetworks are trained adaptively during optimization, and it is possible to retrain only the ones not performing well. As optimization progresses, the subnetworks are adapted to maximize performance near the current search space of the optimization algorithm. The modular neural subnetwork models are combined with an efficient optimization algorithm and are applied to a real coastal aquifer in the Greek island of Santorini. The numerical code SEAWAT was selected for solving the partial differential equations of flow and density dependent transport. The decision variables correspond to pumping rates from 34 wells. The modular subnetwork implementation resulted in significant reduction in CPU time and identified an even better solution than the original numerical model. (C) 2009 Elsevier Ltd. All rights reserved.
Subject Keywords
Coverage
Spatial
Content
Additional Metadata
Name | Value |
---|---|
DOI | 10.1016/j.advwatres.2009.01.001 |
Depth | 100 |
Scale | 11 - 101 km² |
Layers | 10 |
Purpose | Scientific investigation (not related to applied problem);Salt water intrusion |
GroMoPo_ID | 340 |
IsVerified | True |
Model Code | SEAWAT |
Model Link | https://doi.org/10.1016/j.advwatres.2009.01.001 |
Model Time | SS |
Model Year | 2009 |
Model Authors | Kourakos, G; Mantoglou, A |
Model Country | Greece |
Data Available | Report/paper only |
Developer Email | giorgk@gmail.com; mantog@central.ntua.gr |
Dominant Geology | Unsure |
Developer Country | Greece |
Publication Title | Pumping optimization of coastal aquifers based on evolutionary algorithms and surrogate modular neural network models |
Original Developer | No |
Additional Information | |
Integration or Coupling | ANN Surrogate model |
Evaluation or Calibration | Unsure |
Geologic Data Availability | No |
How to Cite
This resource is shared under the Creative Commons Attribution CC BY.
http://creativecommons.org/licenses/by/4.0/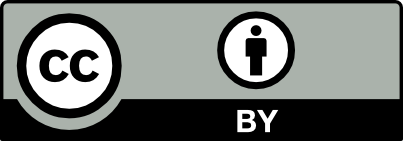
Comments
There are currently no comments
New Comment