Checking for non-preferred file/folder path names (may take a long time depending on the number of files/folders) ...
This resource contains some files/folders that have non-preferred characters in their name. Show non-conforming files/folders.
This resource contains content types with files that need to be updated to match with metadata changes. Show content type files that need updating.
Authors: |
|
|
---|---|---|
Owners: |
|
This resource does not have an owner who is an active HydroShare user. Contact CUAHSI (help@cuahsi.org) for information on this resource. |
Type: | Resource | |
Storage: | The size of this resource is 13.2 KB | |
Created: | May 15, 2022 at 4:39 a.m. | |
Last updated: | May 15, 2022 at 4:56 a.m. | |
Citation: | See how to cite this resource |
Sharing Status: | Public |
---|---|
Views: | 1164 |
Downloads: | 7 |
+1 Votes: | Be the first one to this. |
Comments: | No comments (yet) |
Abstract
Fresh produce irrigated with contaminated water poses a substantial risk to human health. This study evaluated the impact of incorporating sediment information on improving the performance of machine learning models to quantify E. coli level in irrigation water. Field samples were collected from irrigation canals in the Southwest U.S., for which meteorological, chemical, and physical water quality variables as well as three additional flow and sediment properties: the concentration of E. coli in sediment, sediment median size, and bed shear stress. Water quality was classified based on E. coli concentration exceeding two standard levels: 1 E. coli and 126 E. coli colony forming units (CFU) per 100 ml of irrigation water. Two series of features, including (FIS) and excluding (FES) sediment features, were selected using multi-variant filter feature selection. The correlation analysis revealed the inclusion of sediment features improves the correlation with the target standards compared to the models excluding these features. Support vector machine, logistic regression, and ridge classifier were tested in this study. The support vector machine model performed the best for both targeted standards. Besides, incorporating sediment features improved all models’ performance. Therefore, the concentration of E. coli in sediment and bed shear stress are major factors influencing E. coli concentration in irrigation water.
Subject Keywords
Content
Credits
Funding Agencies
This resource was created using funding from the following sources:
Agency Name | Award Title | Award Number |
---|---|---|
Arizona Department of Agriculture | Canal Sediments as Reservoirs of Pathogenic Bacteria in Irrigation Systems | SCBGP19-28 |
How to Cite
This resource is shared under the Creative Commons Attribution CC BY.
http://creativecommons.org/licenses/by/4.0/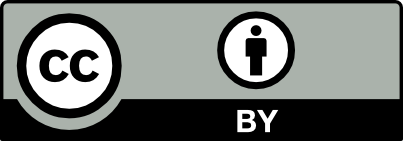
Comments
There are currently no comments
New Comment