Checking for non-preferred file/folder path names (may take a long time depending on the number of files/folders) ...
This resource contains some files/folders that have non-preferred characters in their name. Show non-conforming files/folders.
This resource contains content types with files that need to be updated to match with metadata changes. Show content type files that need updating.
Authors: |
|
|
---|---|---|
Owners: |
|
This resource does not have an owner who is an active HydroShare user. Contact CUAHSI (help@cuahsi.org) for information on this resource. |
Type: | Resource | |
Storage: | The size of this resource is 10.0 MB | |
Created: | Dec 12, 2023 at 6:52 p.m. | |
Last updated: | Dec 12, 2023 at 8:06 p.m. (Metadata update) | |
Published date: | Dec 12, 2023 at 8:06 p.m. | |
DOI: | 10.4211/hs.ad6bdeb1a7f5400193578933cc8a6eae | |
Citation: | See how to cite this resource |
Sharing Status: | Published |
---|---|
Views: | 979 |
Downloads: | 11 |
+1 Votes: | Be the first one to this. |
Comments: | No comments (yet) |
Abstract
Computational hydrology and real world decision-making increasingly rely on simulation-based, multi-scenario analyses. Enabling scientists to align their research with national-scale efforts is necessary to facilitate knowledge transfer and sharing between operational applications and those focused on local or regional water issues. Leveraging existing large-domain datasets with new and innovative modeling practices is vital for improving operational prediction systems. The scale of these large-domain datasets presents significant challenges when applying them at smaller spatial scales, specifically data collection, pre-processing, post-processing, and reproducibly disseminating findings. Given these challenges, we propose a cloud-based data processing and modeling pipeline, leveraging existing open source tools and cloud technologies, to support common hydrologic data analysis and modeling procedures. Through this work we establish a scalable and flexible pattern for enabling efficient data processing and modeling in the cloud using workflows containing both publicly accessible and privately maintained cloud stores. By leveraging modern cloud computing technologies such as Kubernetes, Dask, Argo, and Analysis Ready Cloud Optimized data, we establish a computationally scalable solution that can be deployed for specific scientific studies, research projects, or communities. We present an approach for using large-domain meteorological and hydrologic modeling datasets for local and regional applications using the NOAA National Water Model, the NOAA NextGen Hydrological Modeling Framework, and Parflow. We discuss how this approach can be used to advance our collective understanding of hydrologic processes, creating reusable workflows, and operating on large-scale data in the cloud.
Subject Keywords
Coverage
Temporal
Start Date: | |
---|---|
End Date: |
Content
How to Cite
This resource is shared under the Creative Commons Attribution CC BY.
http://creativecommons.org/licenses/by/4.0/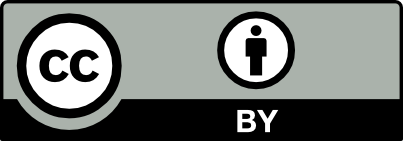
Comments
There are currently no comments
New Comment