Checking for non-preferred file/folder path names (may take a long time depending on the number of files/folders) ...
This resource contains some files/folders that have non-preferred characters in their name. Show non-conforming files/folders.
This resource contains content types with files that need to be updated to match with metadata changes. Show content type files that need updating.
Authors: |
|
|
---|---|---|
Owners: |
|
This resource does not have an owner who is an active HydroShare user. Contact CUAHSI (help@cuahsi.org) for information on this resource. |
Type: | Resource | |
Storage: | The size of this resource is 7.5 MB | |
Created: | Apr 01, 2018 at 4:56 p.m. | |
Last updated: | Apr 09, 2018 at 7:02 p.m. | |
Citation: | See how to cite this resource |
Sharing Status: | Public |
---|---|
Views: | 1465 |
Downloads: | 40 |
+1 Votes: | Be the first one to this. |
Comments: | No comments (yet) |
Abstract
Distributed Temperature Sensing (DTS) technology can collect abundant high resolution river temperature data over space and time to improve development and performance of modeled river temperatures. These data can also identify and quantify ther5 mal variability of micro-habitat that temperature modeling and standard temperature sampling do not capture. This allows researchers and practitioners to bracket uncertainty of daily maximum and minimum temperature that occurs in pools, side channels, or as a result of cool or warm inflows. This is demonstrated in a reach of the Shasta River in Northern California that receives irrigation runoff and inflow from small ground10 water seeps. This approach highlights the influence of air temperature on stream temperatures, and indicates that physically-based numerical models may under-represent this important stream temperature driver. This work suggests DTS datasets improve efforts to simulate stream temperatures and demonstrates the utility of DTS to improve model performance and enhance detailed evaluation of hydrologic processes.
Raw project data is available by contacting ctemps@unr.edu
Subject Keywords
Content
How to Cite
This resource is shared under the Creative Commons Attribution CC BY.
http://creativecommons.org/licenses/by/4.0/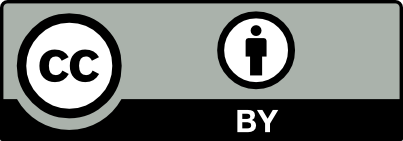
Comments
There are currently no comments
New Comment