Checking for non-preferred file/folder path names (may take a long time depending on the number of files/folders) ...
This resource contains some files/folders that have non-preferred characters in their name. Show non-conforming files/folders.
This resource contains content types with files that need to be updated to match with metadata changes. Show content type files that need updating.
Machine Learning-Based Prediction of Streamflow Volume and Peak Discharge Using Snow, Temperature, and Soil Moisture Data in Logan River, Utah
Authors: |
|
|
---|---|---|
Owners: |
|
This resource does not have an owner who is an active HydroShare user. Contact CUAHSI (help@cuahsi.org) for information on this resource. |
Type: | Resource | |
Storage: | The size of this resource is 1.1 MB | |
Created: | Apr 20, 2025 at 2:03 a.m. | |
Last updated: | Apr 30, 2025 at 7:43 p.m. | |
Citation: | See how to cite this resource | |
Content types: | CSV Content |
Sharing Status: | Public |
---|---|
Views: | 90 |
Downloads: | 0 |
+1 Votes: | Be the first one to this. |
Comments: | No comments (yet) |
Abstract
Accurate prediction of annual streamflow volume and peak discharge is essential for effective water resource management, particularly in snowmelt-dominated watersheds where hydrologic responses are highly sensitive to climate variability. This study applies three regression-based modeling approaches—Multivariate Linear Regression (MLR), k-Nearest Neighbors (KNN), and Random Forest (RF)—to forecast annual streamflow volume and peak discharge using a combination of snow, temperature, and soil moisture data from the Logan River watershed in northern Utah. The input features included maximum snow water equivalent (SWE), average maximum temperature over the three weeks following peak SWE (as a proxy for melt energy), and antecedent soil moisture measured at 2", 8", and 20" depths. The dataset spanned water years 1980 to 2023, with soil moisture data available for 2006 onward. Feature engineering was performed to align predictors with hydrologically relevant timing, such as melt onset and pre-saturation conditions. MLR models using only SWE and temperature achieved R² values of 0.749 for annual streamflow volume and 0.772 for peak discharge, while adding soil moisture improved performance to 0.847 and 0.899, respectively. KNN models using SWE and temperature achieved R² values of 0.804 for volume and 0.776 for peakflow, making it the second-best method when soil data were unavailable. The RF model showed strong performance for peakflow (test R² = 0.746) but was less accurate for volume (R² = 0.646). Across all models, SWE consistently emerged as the most influential predictor, while temperature and soil moisture improved model accuracy by capturing melt energy and runoff potential. These results demonstrate that incorporating multiple hydrometeorological variables improves prediction of streamflow behavior, and that machine learning methods such as KNN and RF offer valuable alternatives to traditional regression, particularly when data availability varies. This framework provides a robust approach to streamflow forecasting under changing climatic and hydrologic conditions.
Subject Keywords
Content
How to Cite
This resource is shared under the Creative Commons Attribution CC BY.
http://creativecommons.org/licenses/by/4.0/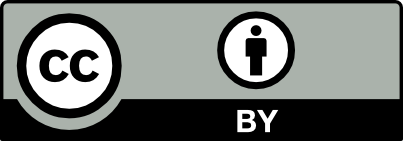
Comments
There are currently no comments
New Comment