Checking for non-preferred file/folder path names (may take a long time depending on the number of files/folders) ...
This resource contains some files/folders that have non-preferred characters in their name. Show non-conforming files/folders.
This resource contains content types with files that need to be updated to match with metadata changes. Show content type files that need updating.
Authors: |
|
|
---|---|---|
Owners: |
|
This resource does not have an owner who is an active HydroShare user. Contact CUAHSI (help@cuahsi.org) for information on this resource. |
Type: | Resource | |
Storage: | The size of this resource is 35.3 MB | |
Created: | May 07, 2025 at 12:47 p.m. (UTC) | |
Last updated: | May 08, 2025 at 1:32 a.m. (UTC) | |
Published date: | May 08, 2025 at 1:32 a.m. (UTC) | |
DOI: | 10.4211/hs.bdc496c09e394875accc732e94daab3b | |
Citation: | See how to cite this resource | |
Content types: | CSV Content |
Sharing Status: | Published |
---|---|
Views: | 235 |
Downloads: | 0 |
+1 Votes: | Be the first one to this. |
Comments: | No comments (yet) |
Abstract
We present a knowledge-guided machine learning framework for operational hydrologic forecasting at the catchment scale. Our approach, a Factorized Hierarchical Neural Network (FHNN), has two main components: inverse and forward models. The inverse model uses observed precipitation, temperature, and streamflow data to generate a representation of the current underlying catchment state. The forward model predicts streamflow using the learned catchment state. The FHNN architecture is designed to model multi-scale processes and capture their interactions, a critical ability for flood modeling. FHNN also improves forecasts based on real-time data through an inference-based data integration approach using inverse modeling. FHNN’s data integration approach improves forecasts in response to observed data more efficiently than data assimilation methods that require computationally intensive optimization. To show the performance of FHNN, we compare the FHNN to a leading deep learning alternative (autoregressive LSTM) on the large-sample CAMELS-US dataset, and operational flood forecast data from the US National Weather Service (NWS). Official NWS flood forecasts are generated by expert human forecasters using a physics-based model, in a human-in-the-loop process. Thus, we assess the flood forecast ability of FHNN by directly comparing its performance against these NWS expert-derived forecasts. The human forecaster creates a more accurate forecast within the first 18 hours of a forecast’s issuance, but FHNN has significantly better predictions thereafter. This research lays the groundwork for leveraging the predictive performance of AI-based models with the expertise in forecasting agencies to produce better river forecasts.
Subject Keywords
Coverage
Spatial
Temporal
Start Date: | |
---|---|
End Date: |
Content
Credits
Funding Agencies
This resource was created using funding from the following sources:
Agency Name | Award Title | Award Number |
---|---|---|
NSF Div. of Information & Intelligent Systems | III: Medium: Advancing Deep Learning for Inverse Modeling | 2313174 |
How to Cite
This resource is shared under the Creative Commons Attribution CC BY.
http://creativecommons.org/licenses/by/4.0/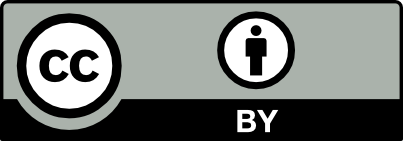
Comments
There are currently no comments
New Comment